While much has been said about the transformative benefits of machine learning models in the insurance industry, less attention has been given to the challenges and complexities of implementation. Asia Insurance Review spoke to Pacific Life Re’s Mr Ong Qian Hao and FWD Group’s Ms Fiona Hermans to understand how strategic partnerships can effectively address these challenges and lead to successful outcomes.
Machine learning certainly is not a new technology. In fact, it was coined sometime in the middle of the 20th century by Alan Turing. It is only in recent years that organisations such as FWD, a pan-Asian life and health insurer with over 12m customers across 10 markets, can leverage the power of machine learning.
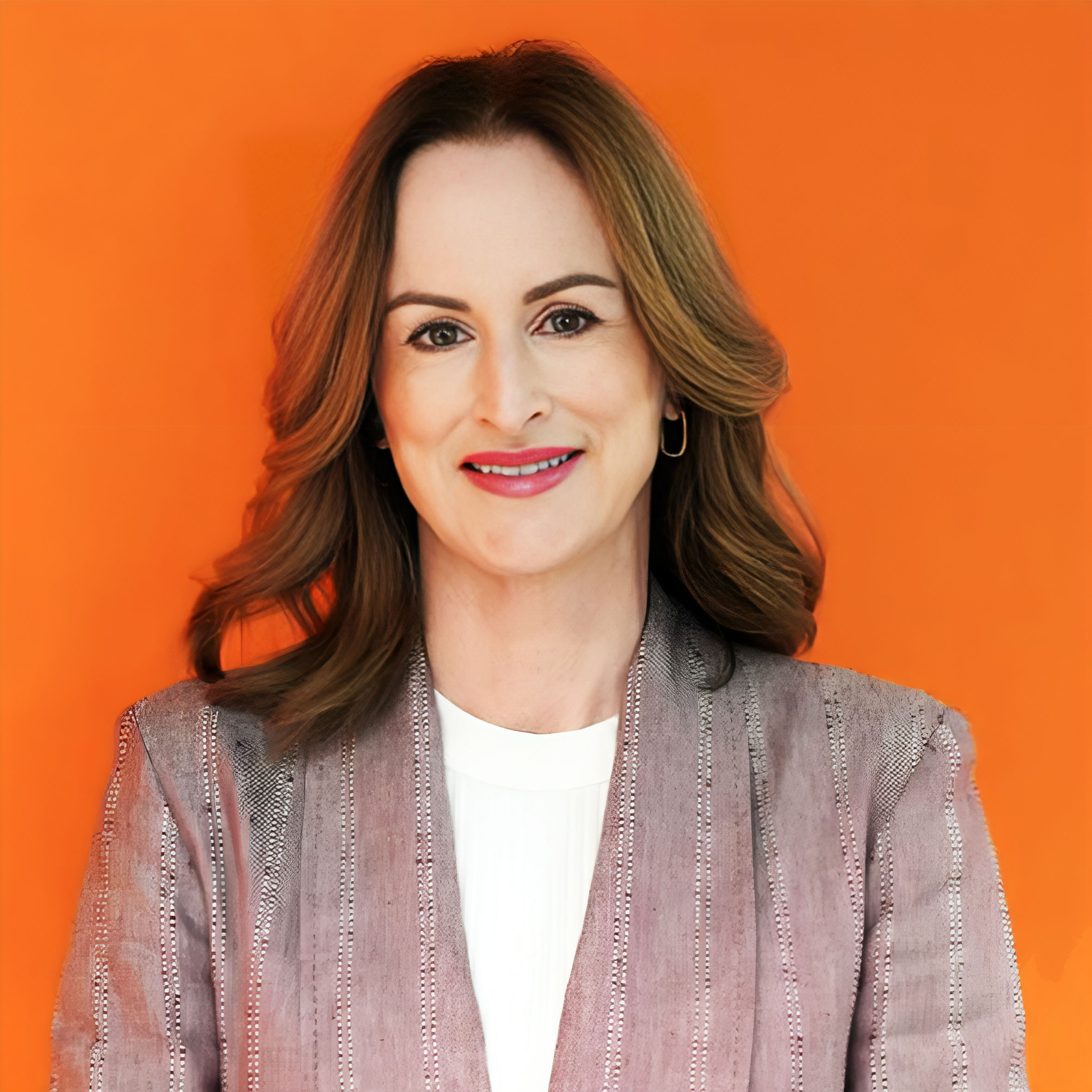
“FWD was born as a customer-led and digitally-enabled company and has been utilising AI, such as machine learning and predictive analytics, across our business since 2019. We recognise the potential for technology to improve customer experience significantly in areas like underwriting by reducing false alerts and questions as one example,” said FWD group head of customer Fiona Hermans.
Pacific Life Re, a global life reinsurer with a presence in over 12 countries across four continents, has been at the forefront of innovation. In the last few years, it has leveraged machine learning and AI to gain deeper insights into both traditional and non-traditional risk factors, improve pricing strategies and help clients deploy their own predictive models.
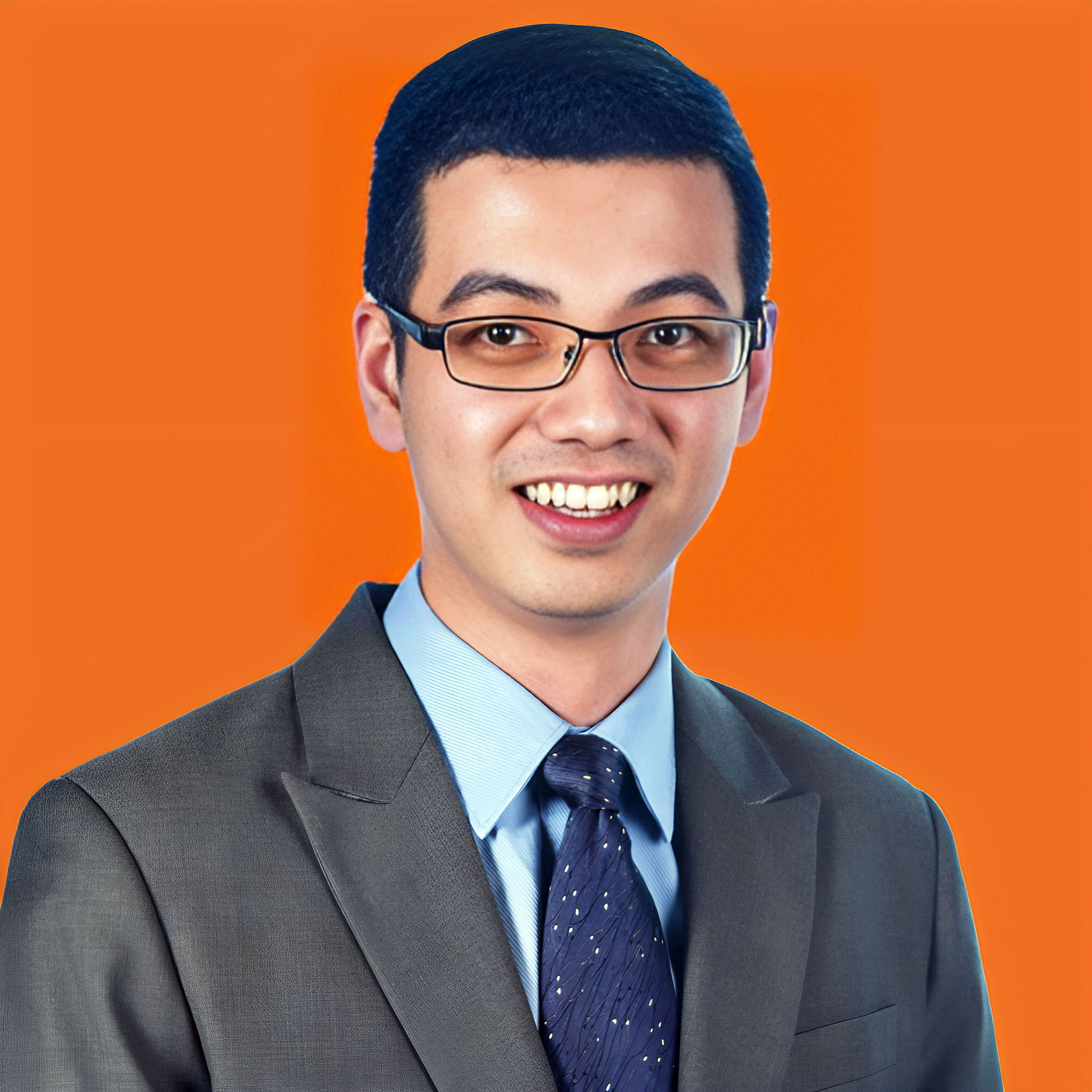
Pacific Life Re global head of strategic analytics Ong Qian Hao said, “While the industry often focuses on the benefits of machine learning, not much attention is given to addressing the technical hurdles and stakeholder management that come with implementing them. Rolling out a successful model usually demands teamwork across different departments and clear communication of technical results to the players.”
The good news? Insurers do not have to embark on this alone. Teaming up with reinsurance partners - who bring broad industry insights and specialised technical expertise - can help speed up and ensure a successful implementation.
An opportunity to innovate: A case study examining underwriting
Most, if not all, insurers have achieved significant efficiencies using automated underwriting systems based on predefined rules and guidelines. Building on this solid foundation, the industry is now at the stage of integrating machine learning into underwriting. Machine learning models can help insurers assess risk more efficiently and yet, still effectively.
This has the potential to accelerate decision-making further, provide a better customer experience with more personalised solutions, and increase overall operational efficiency. This progression represents the next frontier in underwriting, positioning insurers to meet the demands of today’s dynamic market.
The data science team at FWD was keen to deploy machine learning models to support their growing business volumes further. Much work by FWD has gone into internally validating and testing of predictive models, which have been shown to accelerate and streamline existing processes.
FWD partnered with Pacific Life Re who served as an independent expert to assess and quantify the impact on financials and risk exposure of these machine learning models. The underwriting and data science teams from both companies collaborated closely to assess the predictive models, utilising a broad range of stress tests and scenario analyses.
The primary objective was to understand and quantify how these models could impact FWD’s financials and risk whilst ensuring the main objective of the models is met, to improve customer journey and outcomes.
Navigating the challenges of model implementation
Implementing innovative models in the market can pose several unique challenges, primarily because these solutions may be the first-of-its-kind and so lack any precedent, making the adoption a complex endeavour.
Specifically, what is often not discussed is the amount of organisational change required. Stakeholders may be hesitant to embrace unfamiliar technology without proven results or clear benefits.
Questions may include ‘how much faster and better’, ‘can machine learning really help with resource prioritisation and allow underwriters to focus on applications that genuinely need reviewing?’ and ‘what are the risk considerations for such implementations?’. Shifting strategic priorities and resource reallocation often compound this steep learning curve.
Machine learning: Dispelling the myths
Mr Ong said, “Machine learning models are often thought of as a black box. However, the field of explainable AI is growing rapidly and techniques like shapely additive explanations help shed light on how these models operate. Combining these techniques with a range of scenario testing allows stakeholders to understand the impact of deploying such models. This transparency not only builds trust but also enables cost and benefit assessments to be refined and informed decisions to be made.”
Drawing on its extensive market and risk knowledge from its global business, Pacific Life Re’s experienced underwriting professionals, together with its strategic analytics team, provided FWD with an independent and balanced assessment of potential risks associated with deploying predictive models. By combining data science expertise with deep underwriting insights, it utilised a comprehensive range of real-world scenarios - including anti-selection risk and potential model deterioration - to analyse the outputs from the machine learning models and quantify the financial implications.
Mr Ong said, “While machine learning offers remarkable capabilities, it is not infallible. The crucial factor is a deep understanding of the inherent risks, the development of robust mitigation strategies, and the clear communication of these insights to stakeholders. This proactive approach empowers our clients to harness the full potential of machine learning while effectively managing its limitations, ultimately enhancing their decision-making and competitive edge.”
Ms Hermans said, “It’s important to engage the right expertise and advisers to have a comprehensive understanding of the risks of our predictive models from a reinsurance perspective.”
Moving ahead together
As much as new technologies promise a great deal, and that the first movers who leverage it and build products around it will be well positioned to gain market share, the organisational requirements that govern it still present a challenge for many.
“Infrastructure takes time to build and mature, but of equal importance is making sure the right talent and mindset is cultivated for success to be sustainable over time,” said Ms Hermans.
“That’s when strategic partnerships and industry collaboration become truly invaluable in successful deployment of machine learning models,” said Mr Ong.
“By tapping into our global expertise in machine learning, data analytics and underwriting, we want to support our partners regardless of which stage they are at in their digital transformation. Whether it’s offering technical guidance, sharing insights on best practices, or helping to navigate potential risks, we are committed to working collaboratively with them to achieve shared objectives,” he said. A